Richard McClintock, a Latin professor at Hampden-Sydney consectetur adip isicing elit, sed dolore magna aliqua hampden. Latin professor at Hampden-Sydney consectetur.
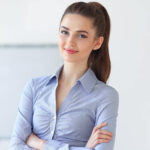
Jack White
CEO“Sed ut perspiciatis unde omnis iste natus error sit voluptatem accusantium incididunt ut labore et dolore Sed ut perspiciatis error. Perspiciatis unde omnis iste.”
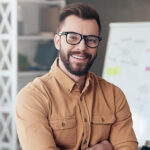
Walhan Bobe
Business Advisor“Contrary to popular belief, Lorem Ipsum is not simply random text. It has roots in a piece of classical Latin literature from 45 BC, making it over 2000 years old.”
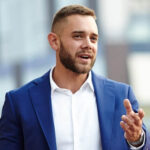
Mark McManus
COO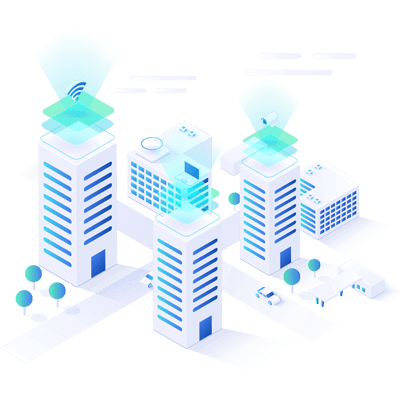
Data Science
A ground-breaking study says 90% of the entirety of the world’s data has been created within the previous two years. In just two years, we have collected and processed 9x the amount of information than the information collected by humankind combined. It’s projected we’ve already created astounding 44 zettabytes of data.
What do we do with all this data? How do we make it useful to us? What are its real-world applications? These questions are the domain of data science.
- one
- two
Pillars of Data Science
While data scientists often come from many different educational and work experience backgrounds, most should be strong in, or in an ideal case be experts in four fundamental areas and these are:
- Business/Domain
- Mathematics (includes statistics and probability)
- Computer science (e.g., software/data architecture and engineering)
- Communication (both written and verbal)
Data Science Goals and Deliverables
To understand the importance of these pillars, one must first understand the typical goals and deliverables associated with data science initiatives, and the data science process. Let’s discuss some common data science goals and deliverables.
Here is a short list of common data science deliverables:- Prediction (predict a value based on inputs)
- Classification (e.g., spam or not spam)
- Recommendations (e.g., Amazon and Netflix recommendations)
- Pattern detection and grouping (e.g., classification without known classes)
- Anomaly detection (e.g., fraud detection)
- Recognition (image, text, audio, video, facial, …)
- Actionable insights (via dashboards, reports, visualizations, …)
- Automated processes and decision-making (e.g., credit card approval)
- Segmentation (e.g., demographic-based marketing)
- Optimization (e.g., risk management)
- Forecasts (e.g., sales and revenue)
The Data Science Process
Data scientists usually follow a process similar to this, especially when creating models using machine learning and related techniques.
In IntelloGrit the Process Model consists of five iterative phases—goals, acquire, build, deliver and optimize. Each phase is iterative because any phase can loop back to one or more phases.